Andrew Jun Lee
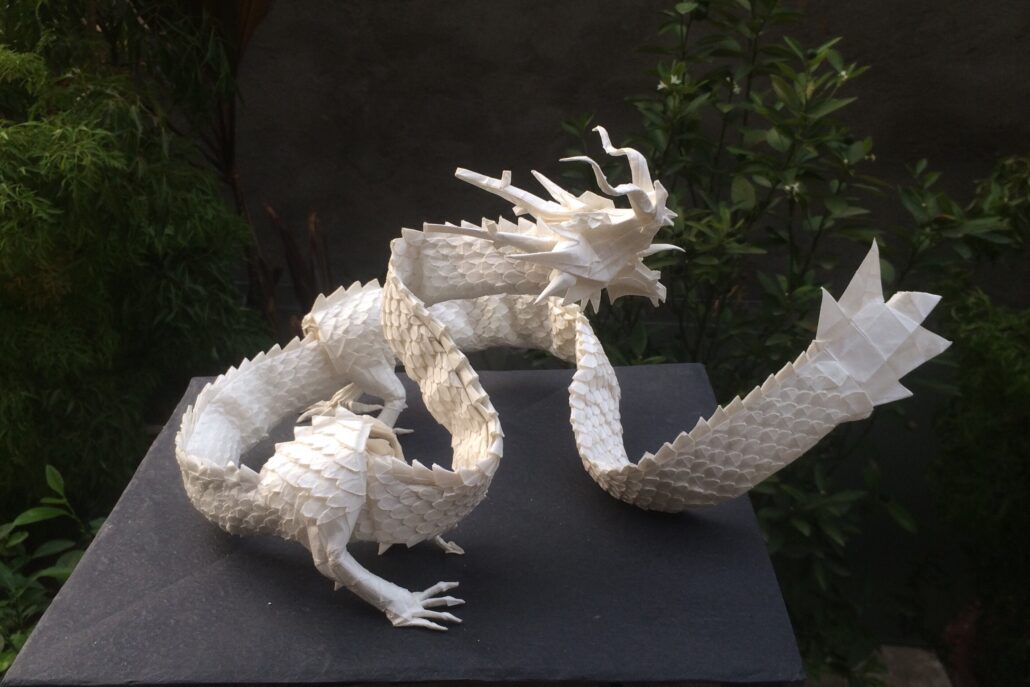
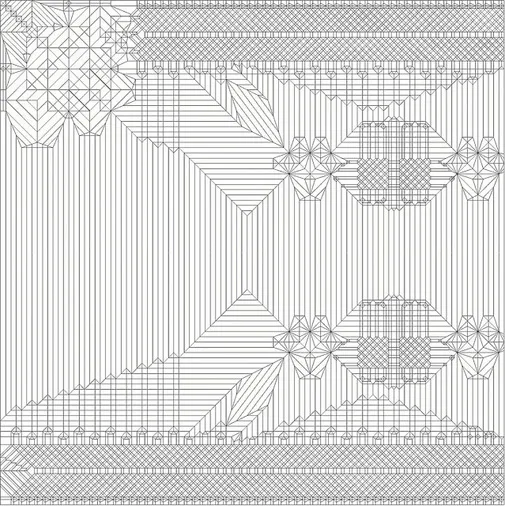
Origami deeply captivated me as a child. On one hand, I was awestruck by the idea that a simple sheet of paper could give rise to dragons, insects, and human figures (Figure 1, Left). On the other hand, I was shocked that unfolding these intricate designs revealed complex arrangements of creases that seemed to hold mystical, but explicable, secrets (Figure 1, Right).
These awestruck feelings came from two directions. From the bottom-up, I blindly followed instructions to make the right creases, somehow ending up with life-like creations. From the top-down, unfolding a finished model led back to a flat sheet of paper, embedded with a complex arrangement of creases; I wondered how these creases related to their three-dimensional form. I’ll argue that both the “forward pass” of origami (i.e., the “engineering” that is folding) and the “backward pass” (i.e., probing complexity from a finished origami model) are roughly reflective of the state of artificial intelligence today.
One part of the analogy is that the mind is like an origami model—borne out of simple tiny units called neurons interacting in complex and unintuitive patterns that bear out the mind. Much like the top-down observer of origami models, neuroscientists and psychologists have uncovered that this complexity consists of simple neuronal units (corresponding to the creases in origami), and that the prize in the pudding is what their interactions mean (corresponding to the overall patterns of creases in origami).
But the analogy goes deeper—obscurity also lies in both directions. The engineer of complex neural networks has a broad understanding of how these networks work, but not the network’s states that emerge after training. Similarly, the experimenter of neural networks seeks to obtain a concrete understanding of these emergent states. Yet, there is irony for the engineer, the experimenter, and the origami folder: all have the requisite information to attain their goals (e.g., folding an origami model and training an intelligent machine), but the act of folding paper and training neural networks still elude us from their underlying secrets.
The Large Language Models of today, such as chatGPT and Bing Chat, are examples of this ambiguity. In the same year that GPT-3 (i.e., chatGPT’s “parent” system) was unveiled to the media (with a shocking self-written article about itself) (GPT-3, 2020), academic papers were being published trying to answer what models of a simpler architecture were exactly doing (Rogers, 2021). These papers seek to understand what “small” language models like BERT have learned from training (e.g., the emergent patterns in an unfolded origami model). Models like BERT are fundamentally similar in architecture to models like GPT. Again, the irony is that academia is capable of creating incredible zeitgeist-changing machines whose inner workings we do not grasp.
In short, interpretation plays a secondary role in designing ever more intelligent machines. This is one major point of difference with origami. Origami artists like Robert J. Lang and Satoshi Kamiya broadly understand how the creases of a sheet of paper collectively create the head, tail, antennae, legs, and wings of an insect. Their understanding is informed by various computational tools, such as Lang’s TreeMaker, which pre-determine how the paper comes together from its two-dimensional creases (Lang, 2015). Mathematical theorems also link what we see on paper and the three-dimensional properties of the final origami model (Lang, 2012). These theorems and computational tools are roughly descriptive of what cognitive science lacks: a conceptual bridge between sets of neurons and emergent phenomena, like categorization, memory, and problem-solving.
Take, for example, the circle-packing theorem in origami. This theorem asserts that areas of intersecting creases in a flat sheet of paper, called “circles,” are the motifs that form three-dimensional structures called “flaps.” In origami, a flap is any part of the paper that protrudes from the body of the paper. For example, the traditional paper plane has two large flaps that form the wings; the origami crane has four flaps that constitute the head, the tail, and the two wings. Each of these flaps, according to circle-packing, correspond to “circles” (i.e., regions of intersecting lines) in the flattened sheet of paper.
This insight was influential to origami design because, if an origami designer wanted to add two antennae to an origami model of an insect, this meant that the designer had to add two additional “circles” onto the flat sheet of paper (Lang, 2012). No longer would a tedious process of guess-and-checking be necessary; something more principled, relating different representations of an origami design, could inform origami designers of three-dimensional outcomes from two-dimensional imprints.
But perhaps the right way to frame why it was important is not in terms of practical use, but in terms of “ontological” revision: the idea of a circle is an emergent property of a collection of simpler units (i.e., creases), and is the right level of description underlying three-dimensional form. By re-framing sets of intersecting creases as singular units of circles, the origami designer has re-represented the basic terminology of origami: instead of speaking in terms of lines and creases, the relevant terms of origami design have become circles (as well as adjacent concepts like box-pleats). Notice that these terms supersede any particular line or crease.
It would be useful to achieve a similar theoretical advance in cognitive science, a dearth that grows more worrisome with ever unpredictable behavior in Large Language Models (Roose, 2023). This theoretical advance would be one of “ontological” revision: rather than discussing what sets of neurons are doing in a neural network, it would be helpful to understand what properties they collectively give rise to (e.g., like circles). Revising ontology is not new to cognitive science. In the same way that the birth of cognitive science involved a re-working of folk psychological constructs like beliefs, intentions, desires, emotions, and thoughts into lower-level constructs of algorithms, computations, mechanisms, and processes, so again should ontology be re-worked into terms that bode closer to the realm of neuroscience—should such a re-working be possible in the first place.
This is one way of saying that levels of analysis in cognitive science should be extended beyond the traditional three that David Marr (1982) sketched out in his book Vision (i.e., the computational, algorithmic, and implementational levels). In particular, I think that the algorithmic level should be expanded, though there are substantial questions about whether neural networks are amenable to such “explanatory decomposition” (Bechtel & Richardson, 1993; Love, 2021). If origami is any guide, then what such an expanded list of levels of analysis might include are ontological links between algorithmic outcomes (i.e., three-dimensional flaps/shapes/forms) and sets of neurons (i.e., two-dimensional arrangements of creases).
My belief is that new constructs will help us understand what “black-boxes” like Large Language Models are doing. However, understanding what such constructs would look like, or whether forming them is possible at all, are precisely the difficulties of this. Perhaps, in the future, origami will become not just an analogy of such theoretical fortune, but also an informative guide.
References
Bechtel, W., & Richardson, R. C. (1993). Discovering complexity: Decomposition and localization as strategies in scientific research. Princeton University Press.
GPT-3. (2020, September 8). A robot wrote this entire article. Are you scared yet, human?. The Guardian. https://www.theguardian.com/commentisfree/2020/sep/08/robot-wrote-this-article-gpt-3
Lang, R. J. (2012). Origami Design Secrets: Mathematical Methods for an Ancient Art. CRC Press.
Lang, R. J. (2015, September 19). TreeMaker. Robert J. Lang Origami. https://langorigami.com/article/treemaker/
Love, B. C. (2021). Levels of biological plausibility. Philosophical Transactions of the Royal Society B, 376(1815), 20190632.
Marr, D. (1982). Vision: A Computational Investigation into the Human Representation and Processing of Visual Information. W. H. Freeman and Company.
Rogers, A., Kovaleva, O., & Rumshisky, A. (2021). A primer in BERTology: What we know about how BERT works. Transactions of the Association for Computational Linguistics, 8, 842-866.
Roose, K. (2023, February 17). A Conversation With Bing’s Chatbot Left Me Deeply Unsettled. The New York Times. https://www.nytimes.com/2023/02/16/technology/bing-chatbot-microsoft-chatgpt.html